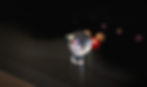
In a prior post, we talked about a way to think about getting better at hiring. I teed up a notion of WHAT HOW and WHO as the levers that you can use to drive better accuracy in hiring.
I also noted that you need to match the gain in accuracy vs the value of the data vs the need to get it right.
I wanted to blow that out a bit more to be tactical. I'm sure there are better and more accurate lists…but here's some general guidance on how to think about this. For the typical senior executive, I have ordered WHAT WHO and HOW from top to bottom roughly on the ones that have the best chance of giving you good data. I reserve the right to change my mind for the order. Also (and unfortunately) many of the items in the lists (especially HOW) also are ordered from time they take and money it costs from top to bottom. There is no free lunch. I’ve suggested the investment costs (a combo of time and money) of each item with ++ signs.
WHAT
1. +++ Experience (the mantra here is past performance is the best predictor of future success)
2. ++ Abilities (this is the psych stuff--probably better at predicting future potential)
3. ++ Skills (what they bring to the table--probably better at predicting current performance)
4. + Knowledge (what they know, but can frequently be taught or learned)
HOW
1. ++ Personal observation (your own experience on what they've done and how they've done it, ideally from direct contact in the workplace)
2. ++++ Structured Behavioral Interviews (the gold standard)
3. +++ References (especially back door)
4. ++ Data-driven decision meetings against a clear job description (this isn’t quite the same, but don’t underestimate the value of taking all the great data that you collect and making a decision in a thoughtful way)
5. ++ Sourcing from friends/people you trust (also a little different, but you are replacing your own screening with someone else's observations and judgment on the fit. For some, this is a great approach)
6. ++ Prior Performance reviews (especially if the role is similar)
7. +++ Assessment centers
8. ++++ Search firm screening (The caveat, as always, is understand how the economics and incentives are structured so you know the inherent biases)
9. +++ Psych profiles (there are a lot of individual psychologists who will screen people for you)
10. +++ Personality tests (e.g., Hogan, Caliper, Good.co)
11. +++ Intelligence tests (e.g., Wonderlic, Watson-Glaser, Raven's)
12. +++ Panel interviews (These can be done well, but they typically are more stilted and run the risk of group think)
13. ++ Work samples (The biggest risk is that you over-interpret what you see as a reflection of how the person will operate)
14. + Random and Unprepared Interviews (I include this as a way to position the most common interview, conducted with all the pitfalls that give rise to a 50% success rate)
15. +++ Public source investigations (I have heard some good things about this, but don't have enough experience to be confident in the quality)
16. + Applications, Resumes and/or LinkedIn (There are ways to get insights, but it takes some work and a lot of practice to see through the fluff)
17. + Social media profiles
18. + Gut feel (To be fair, I do use my gut. You should too. But I also evaluate my biases and never allow my gut to trump actual data.)
WHO
1. +++ Manager (prepared and well-trained)
2. ++++ 3rd party professional
3. ++ HR professional
4. ++ Someone you trust
5. + Manager (unprepared)
6. + Your significant other (I’m not suggesting that your significant other isn’t insightful, just that they are not always judging on the criteria that’s most relevant. That being said, after a year of working with someone, your significant other probably CAN tell you whether it was the wrong hire...)
? +++ Algorithm/AI (I think there is potential here, and my evaluation may change as I read more about it, but every algorithm gets written by a human being who has a data set that they train the algorithm on…and you have to think about and pressure test and question whether the test data set is a good, fair, and representative sample and the people who wrote the algorithm have any implicit biases that they have enshrined in the code)
An example
For sake of example, say you are hiring 5 sales people a year. Your overall turnover has thus far been nearly 75% per year, which is unacceptably high, especially since it means that you’re spending too much time onboarding and training new people all the time. And all of that investment just goes down the drain when they leave. You don’t have an unlimited budget, but you need to get better. So based on some careful consideration, you decide to make some change to go…
FROM
Sourcing by job boards
3 unstructured interviews by whoever is in the office
Decision made by a manager who JUST NEEDS SOMEONE YESTERDAY
TO
Referrals from your network
Online testing for candidate psych profile, compared to successful sales profiles
2 structured interviews, one of which is done by the best interviewer in HR
3 reference calls
A consistent decision meeting, complete with tracking results over time and yearly look-backs on success
In this case, you’ve bent the curve, haven’t spent that much more time than you did before (you’ve eliminated 1 interview and replaced it with reference calls and a structured decision meeting). You might spend a little more money training people. But most importantly, you increase your accuracy and confidence from 50% to 75%. Increasing your accuracy means that you’re going to have to hire fewer people every year and get better results. Seems pretty good.